
The pace of innovation in the medical field is constantly accelerating, which means a greater understanding of human health conditions and the possibility of more effective treatments. However, identifying a condition, molecule, or therapeutic target doesn’t automatically mean we can cure a disease. The human body is complex, and each new discovery reveals more questions about how to develop the right therapies.
Fortunately, technology can support faster identification of scientific breakthroughs. At CAS, we applied machine learning algorithms to develop predictive models that can assist in finding effective new drugs to treat many diseases including cancer, viral infections, and inflammatory disorders. We focused on TBK1 (TANK-binding kinase 1), an enzyme with kinase activity that plays a crucial role in multiple biological processes. Targeting TBK1 has the potential to drive new therapies for life-threatening diseases, yet its complexity presents research challenges.
We analyzed the CAS Content CollectionTM, the largest human-curated repository of scientific information, and found that journal publications on TBK1 have nearly doubled between 2020 and 2023. This suggests extensive interest in the research community, but the low growth we’ve seen in patent publications speaks to the potential difficulties in targeting TBK1.
Because TBK1 plays a critical role in immune and cellular responses, it’s a very promising target for new drug therapies, and researchers need tools to overcome the complexities related to this kinase and find breakthroughs faster. Our computer modeling approach shows how it can be done.
TBK1 plays a role in cancer, autoimmune diseases, and more
Kinases are enzymes that catalyze the transfer of a phosphate group from a high-energy molecule, such as ATP, to a specific substrate molecule, typically a protein, lipid, or carbohydrate. Kinases are essential for transmitting signals within cells and external stimuli. They’re involved in many physiological processes. As an important kinase, TBK1 is:
- A key regulator of the innate immune response to viral and bacterial infections.
- Involved in the regulation of inflammatory responses.
- Important for the regulation of autophagy, a cellular process involved in the degradation and recycling of damaged organelles and proteins.
- A contributor to cell survival and proliferation in various contexts.
- Involved in the regulation of metabolic processes, including glucose metabolism and lipid homeostasis.
- Implicated in the cellular response to DNA damage.
Because of its importance in so many biological processes, TBK1 has been implicated in various diseases. In our analysis of the CAS Content Collection, we visualized the co-occurrences of TBK1 with other proteins and diseases in scientific literature over the last 20 years (see Figure 1). Notable disease co-occurrences include:
- Autoimmune diseases such as lupus, multiple sclerosis, and irritable bowel syndrome.
- Neurodegenerative disorders like ALS and frontotemporal dementia.
- Cancer development and progression, where TBK1 can enhance cell proliferation, inhibit apoptosis, and modulate the tumor microenvironment in ways that cause tumor progression.
- Viral infections, which may be impacted by TBK1 dysregulation affecting the immune system’s response to viruses.
- Metabolic disorders, but the precise mechanisms are still being explored.
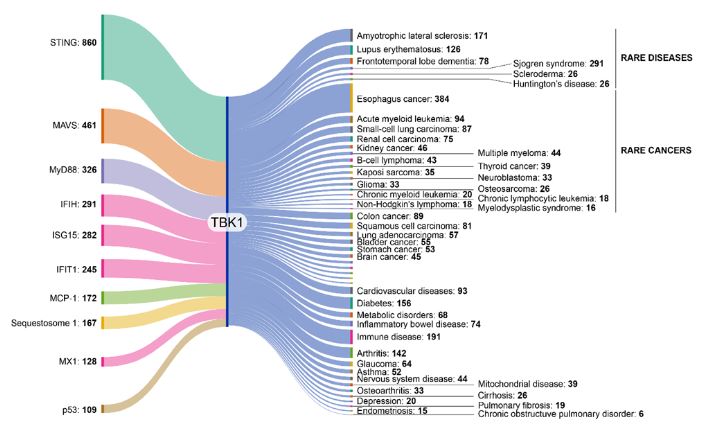
Researchers are pursuing TBK1 inhibitors that can selectively block this kinase’s activity. Targeting TBK1 in conditions where it’s dysregulated or overactive could result in significant clinical breakthroughs, including for cancer treatment. Despite promising preclinical results, the development of TBK1 inhibitors faces several challenges, including achieving sufficient selectivity to minimize off-target effects, optimizing pharmacokinetic properties for effective delivery and distribution in the body, and ensuring safety and tolerability in clinical settings.
QSAR modeling identifies potential therapies based on IC50 values
When developing an inhibitor drug, researchers examine the IC50 (half-maximal inhibitory concentration) value. IC50 is a measure that quantifies the potency of an inhibitor, particularly in enzyme inhibition studies. It represents the concentration of an inhibitor required to inhibit 50% of the activity of a biological or biochemical target, such as an enzyme or a cellular process. IC50 is the most widely used and informative measure of a drug's efficacy.
In computer-aided drug design, predicting the IC50 values of potential drug candidates is crucial for assessing their potency in inhibiting the target enzyme or protein. Quantitative structure-activity relationship (QSAR) models are useful for making these predictions because they relate the chemical structure of compounds to their biological activity, including IC50 values. By analyzing a dataset of known inhibitors with experimental IC50 values, QSAR models can predict the IC50 values of new compounds.
To accomplish this, we used the CAS Content Collection to extract all available data on IC50, pIC50, and TBK1. After some data scrubbing, we arrived at a training set of 1,183 compounds. About 40% (475) of them were represented with exact measurements of IC50, which is suitable for developing regression models. The rest of the data was represented as binary (active/inactive) at two different breakpoints: IC50=1uM and IC50=0.1uM. These were suitable for developing binary distribution and three-category classifier models.
Because scientists and regulatory agencies have different needs for predictive capabilities, we chose to build these three different model types.
Fragment-functional analysis descriptors drive the best results
When developing our models, we used three different sets of molecular descriptors: proprietary CAS Fingerprint, available descriptors in RdKit, and fragment-functional analysis.
Of these, we found that the best overall predictive models were derived from the fragment-functional analysis descriptors (see Figure 2). For this analysis, over 66,000 single fragments were generated after splitting the chemical structures of the training set into sub-fragments. We performed this analysis on all three model types (regression, binary distribution, and three-category classifiers).



We then utilized machine learning algorithms to determine the importance of particular molecular descriptors (see Table 1). Feature selection is a critical part of any machine learning technique. It aims to extract the most informative features and remove noise as well as irrelevant and redundant attributes. Table 1 shows the top active molecular descriptors from the fragment-functional analysis that are mainly present in the most potent TBK1 inhibitors.
The predictions for new molecules obtained from a QSAR model are acceptable only if the new molecules fall inside its applicability domain (the chemical space defined by all molecular descriptors used to build the model). Figure 3 shows the Williams plot that represents the applicability domain as a plot of Leverage (h) vs. Standardized Residuals (σ). The applicability domain of our model is defined within ±3σ and a warning leverage threshold h* = 0.25.
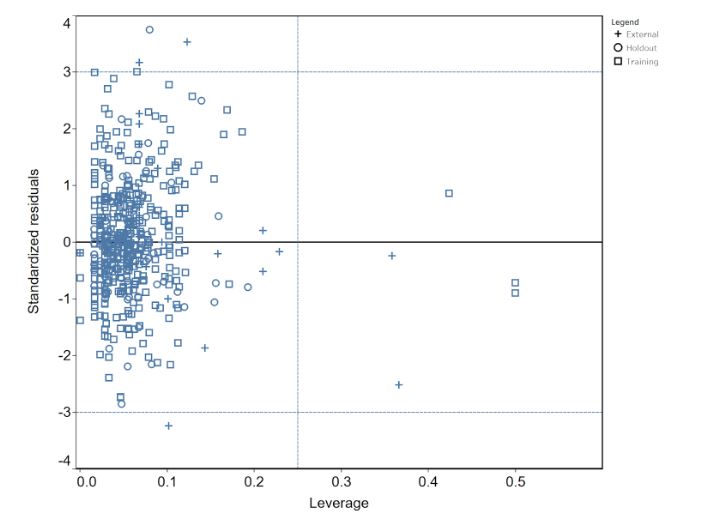
This analysis demonstrates that machine learning tools and computer modeling can indeed help researchers identify promising drug therapies from complex data sets. By applying various modeling approaches, we can identify potential targets for TBK1, which can drive the development of drugs to treat cancer, neurodegenerative diseases, autoimmune conditions, and more conditions as medical discoveries continue.
Analyzing vast amounts of data and accounting for the complexity of compounds and molecules is possible. By using cutting-edge technology to synthesize that data into predictions, we can reach new breakthroughs in drug discovery.
To learn more about the exciting and dynamic field of TBK1 inhibitors and predictive model building, read our new CAS Insights Report, and our recent article in the “Journal of Chemical Information and Modeling.”