
Natural products are compounds, substances, or mixtures produced by plants, animals, microbes, and other members of the natural world. For millennia, people have used natural products to cure all sorts of ailments and, before the advent of modern medicine, were humanity’s only pharmaceuticals.
According to the World Health Organization (WHO), around 80% of the world’s population today is estimated to use traditional medicine. In the past 50 years, natural products and their derivatives have still provided sources of new drugs, but there are major challenges to the large-scale usage of these products due to their insufficient bioavailability and complicated chemical synthesis.
With the advent of advanced computing, better data storage facilities, sophisticated natural language processing techniques, and machine learning (ML)-based capabilities, researchers now have powerful new tools at their disposal to incorporate into studying natural products. Artificial intelligence (AI) is making new advances possible, and medical science has the opportunity to continue leveraging the best of what nature has to offer to cure human ailments.
Recent advances in AI-driven research
We examined data from the CAS Content CollectionTM, the largest human-curated collection of published scientific information, to map the recent publication landscape (2010 onwards) of AI in the field of natural products. With our bird’s eye view of the world’s published science, we found that AI has made significant progress recently in areas like structure prediction, data integration, and more with natural products that are accelerating drug discovery.
Our analysis found over 600,000 scientific publications (including journal articles and patent publications) related to natural product research since 2010. Journal publications dominate the field, and the ratio of patents to journals has decreased in the last few years, indicating an increased interest in academic research over commercial products. How does AI fit into this research? We’ve noted various areas of natural product research where AI, machine learning algorithms, and neural network-based studies are making an impact:
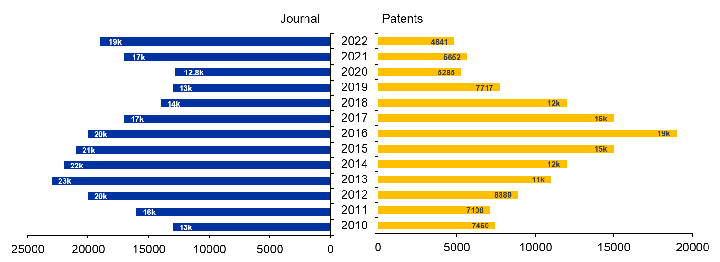
- Compound/Target identification: AI, with the support of machine learning algorithms, can analyze spectroscopic data to identify and characterize compounds present in natural products. This expedites the process of identifying and isolating bioactive molecules. For instance, a highly cited article published in Nucleic Acids Research describes a web server, NRPSpredictor2, which uses machine learning methodology for improved substrate specificity predictions of natural product biosynthetic enzymes in bacteria. Plants and microbes produce natural products as secondary metabolites using genes known as biosynthetic gene clusters (BGCs). AI is being used to predict BCGs that may encode for these metabolites.
- Drug discovery: AI and its subfields such as machine learning are being applied at different stages of the drug discovery pipeline. For instance, AI models are used for the virtual screening of natural product databases, predicting potential drug candidates, and assessing their pharmacological properties. Deep neural networks (DNNs) are key to these efforts, and AI-based generative models can predict drug candidates and accelerate the drug discovery pipeline by narrowing down the number of compounds for experimental validation.
- Bioactivity prediction: Machine learning models can predict and rank the biological activities of natural products from chemical structures using deep neural network-based 3D pharmacophore matching approaches, referred to as quantitative structure–activity/property relationship (QSA/PR) models. These models can help identify compounds with specific therapeutic potentials. In a recent study, machine learning-based methods were used to perform in silico predictions for antibiotics against Acinetobacter baumannii, eventually leading to the discovery of Abaucin which has a bactericidal activity against A.baumanni. In another study, an AI-based approach using a bacterial, natural, product-trained dataset helped discover the antibiotic role of Halicin.
- Optimization of extraction processes: AI can assist in optimizing extraction parameters for obtaining maximum yields of bioactive compounds from natural sources. This reduces the time and resources needed for testing drug candidates.
- Data integration and analysis: AI facilitates the integration and analysis of vast amounts of data from genomics, proteomics, and metabolomics studies. This holistic approach enables a better understanding of the complex interactions within natural systems.
- Predicting synergies: AI tools can predict synergistic interactions between different compounds, guiding researchers in formulating combination therapies using natural products. This is particularly valuable in addressing complex diseases.
- Toxicity prediction: AI models can predict the potential toxicity of natural compounds, ensuring the safety of these products before they are developed into pharmaceuticals or health supplements.
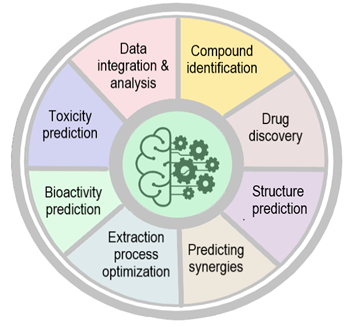
Interest in AI and natural product research has been growing quickly in recent years with 650 journal and patent publications and a corresponding increase in patent-to-journal ratios, which indicates greater commercial interest. While this is a relatively small number of publications, publications have steadily increased from 2010-2022, with a spike since 2020 (Figure 3). We found that China dominates the publication landscape, followed by the U.S. and India, which correlates to the prevalent use of natural products in Chinese traditional medicine and the introduction of the New Generation Artificial Intelligence Development Plan of China (2015–2030), which aims to develop AI-related capabilities in China.
Interest is also growing worldwide — we noted organizations publishing on this topic in Germany, Brazil, South Korea, Germany, the UK, Portugal, Poland, and more. The drug discovery efforts being researched also cover a range of possibilities.
Opportunities for AI in drug discovery
AI can play a role in the identification, classification, and activity prediction of natural products. Plants are a known source of various bioactive secondary metabolites, such as alkaloids and flavonoids, with antiviral, anticancer, antibacterial, and antifungal properties. AI-powered programs and technologies can review and analyze natural products for these properties at a faster pace and assimilate data efficiently - thereby predicting biological activity and speeding up the drug discovery process.
For example, different species of fungi (mushrooms) have been explored for their anti-cancer, immune-modulating, anti-neurodegenerative, anti-inflammatory, and antioxidant properties. AI and ML-based algorithms can be used to classify novel mushroom species and identify their natural products using image-based recognition, devise strategies for optimizing natural product extraction from fungi, and map new uses and properties of different mushroom or other fungal species (Figure 5).
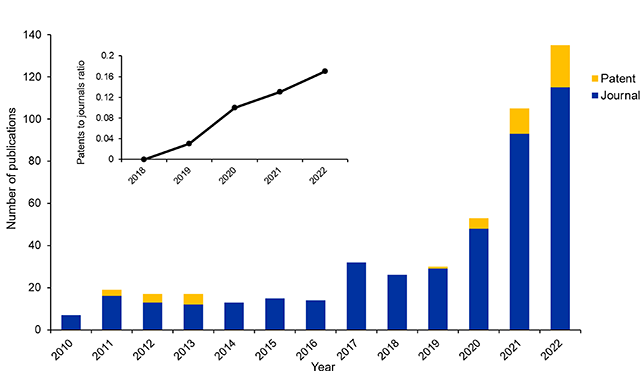
The current landscape of AI and natural products
The most common AI application in natural products today is in anti-tumor agents (Figure 4A) followed by antiviral and antibacterial agents. Analgesics (pain-relieving medications), which constitute a small percentage (2%) of overall top applications, have shown a five-fold percent increase in the number of documents from 2021-2022 (Figure 4B). Other application categories that have shown rapid growth are anti-inflammatory agents, antidiabetic agents, anti-neurodegeneration, and antimalarial agents. Interestingly, the percentage of documents associated with antibacterial agents has reduced from 2021 to 2022, indicating a decreased interest of the scientific community in this field.


We performed substance data analysis leveraging the CAS Content Collection and found about 5,000 substances that co-occur with AI in natural product research in journals and patent publications from 2010-2022 (Figure 6A). Further investigation into the classes of substances suggests that organic and inorganic small molecules, protein/peptide sequences, polymers, elements, and salts are the most important. The number of substances classified as organic/inorganic small molecules is nearly 60 times higher than the next class of substances, protein/peptide sequences, and elements.
Among organic/inorganic small molecules, quercetin shows the highest level of co-occurrence with AI use. Quercetin is a bioactive plant flavanol with powerful antioxidant and anti-inflammatory properties. It has shown potential in the treatment of cancer, AIDS, hypertension, and diabetes. Recently, quercetin, along with kaempferol (another small molecule that shows high co-occurrence with AI use), has demonstrated a positive effect against the COVID-19 virus. AI is being utilized for designing models to help optimize the extraction of quercetin from plant sources, designing novel quercetin analogs, and creating models for testing its anti-oxidative and anti-cancer effects.
Upon closer inspection of proteins/peptide sequences, vancomycin shows maximum co-occurrence with AI, especially for designing studies involving dosage titrations to find optimal dosage levels. Similarly, ML approaches are used to model cyclosporin concentration in renal transplant models. In the polymer category, chitosan shows the highest levels of co-occurrence with AI, which correlates with the studies highlighting AI-based synthesis and testing of chitosan nanoparticles for antimicrobial applications.
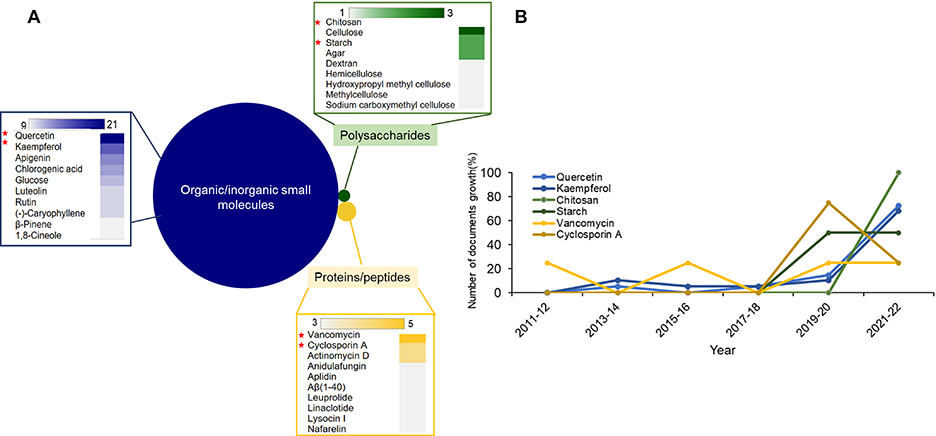
Future outlook and opportunities
The past decade has been revolutionary for AI use in drug discovery, and the natural products field is no exception. AI has gone from being used for the digitization of natural product information to ML-based algorithms providing bioactivity predictions, to recent studies where scientists used neural networks for genome mining and designing natural product-inspired molecules. Other sub-branches of AI, such as BioNLP, which is based on algorithms that contain widespread medical representation, can even be used to extract information from scientific publications to identify newer bioactive plants or natural product sources.
AI has led to a paradigm shift in natural product research, but certain challenges remain. One of these is dereplication, where the same compounds or molecules are being discovered repeatedly. This problem could be mitigated using sophisticated AI-powered databases and tools. Another challenge with natural products is that they’re often discovered without known protein targets. In such cases, AI can help by predicting their targets.
Integration of AI into natural product research is still in the nascent stages, and it’s important that predictive models are fully trained to identify and categorize novel natural products. As this research continues, publication trends indicate that AI will become more extensive in various stages of natural product research. The opportunities for finding new drug molecules from natural sources will continue to grow, and the pharmaceutical pipeline — and, ultimately, patients — will benefit. Learn more about our recent work with expanding the NuBBE database in Brazil and the impact of better data to improve AI predictions.