
Over the last 20 years, advances in artificial intelligence (AI), specifically machine learning, have transformed the way we approach scientific research. From mapping genome sequences and discovering new antibiotics, to modeling the impacts of climate change on Earth, and even mapping the galaxy in the search for other earth-like planets, AI is transforming research across a multitude of disciplines.
Chemistry is one such area of science making huge leaps in the adoption of AI. Our latest whitepaper, "Artificial Intelligence in Chemistry: Current Landscape and Future Opportunities", explores the connection between AI and chemistry using our own technologies to map the publication and patent landscapes. We have uncovered the areas of chemistry that are leading the field with AI and those with great potential yet to be unlocked by the adoption of AI technology.
Where has AI in chemistry grown?
The number of chemistry publications and patents involving AI has exploded, with a six-fold increase observed in the period from 2015 to 2020. We have identified the major disciplines contributing to AI-related publications and patents, and compared them to understand which areas are capitalizing on this emerging technology. Disciplines leading in AI adoption include analytical chemistry, biochemistry, and industrial chemistry and chemical engineering, while areas with an opportunity for AI adoption include natural products and organic chemistry (Figure 1).
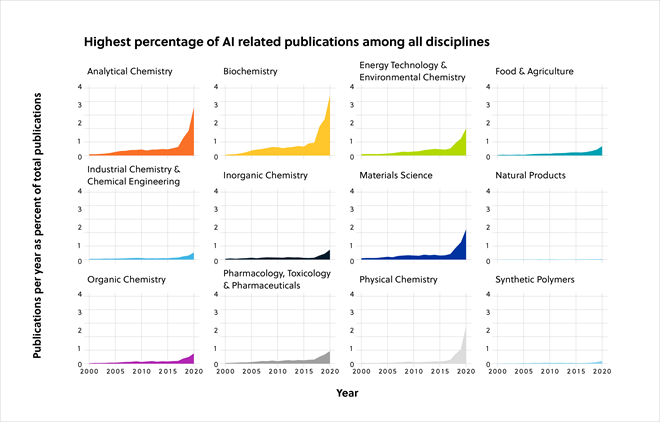
We explored the relationships between these publications and patents from 2000 to 2020 to understand how using AI has helped researchers solve problems (Figure 2). For example, between the early 2000s and 2014, the focus of AI publications and patents shifted from exploring disease diagnoses in humans to genetic algorithms and applying these to drug discovery and microRNA.
More recently, as the types of problems requiring solutions have changed, publications and patents have shifted more towards DNA methylation and cancer. Even more recently, the focus has trended towards drug discovery related to COVID-19.
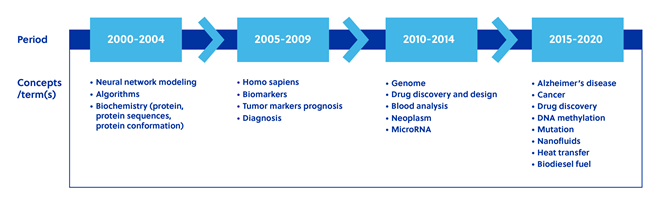
Not surprisingly, our research also identified that small molecules were the biggest focus of the AI publications and patents analyzed. These encompass topics in drug discovery, retrosynthesis, and reaction optimization, reflecting where there is typically more investment from pharmaceutical companies.
Where are the opportunities for machine learning in chemistry?
In our analysis of more than 70,000 publications, we examined interdisciplinary contributions, noting primary and secondary disciplines (Figure 3). This allowed us to plot every discipline onto a heat map, on which the color intensity reflects the strength of contribution for each discipline. At a glance, we can see the areas of study within chemistry that are leading the way with AI and those with unrealized potential.
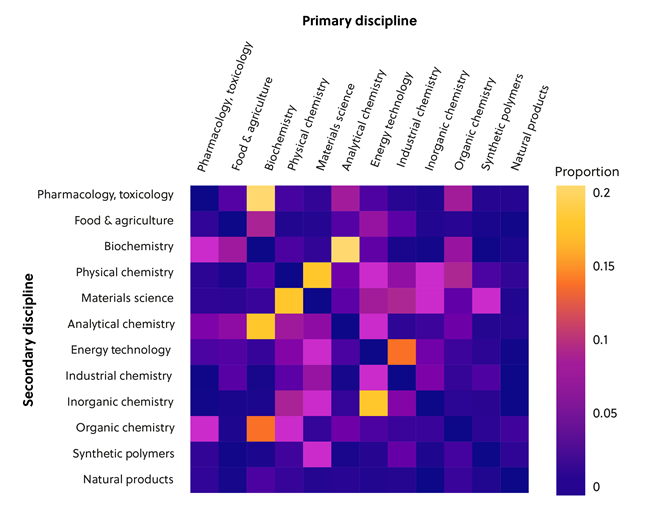
For example, multidisciplinary publications are more common in analytical chemistry and biochemistry, where machine learning algorithms are being used to improve analysis of proteins, peptides, lipids, and nucleic acids, as well as predict chemical reactions or even discover new molecules. AI is also being widely used in materials science and physical chemistry, where the two disciplines are aiming to predict functional materials, structure-property relationships, and chemical process optimization.
The barriers to adopting AI in chemistry
Leading experts discussed the potential barriers to the adoption of AI in our webinar, Artificial Intelligence in Chemistry: Current Trends and Future Opportunities. They identified three key barriers to adopting AI in chemistry:
Data quality: Optimal predictions are dependent upon robust, high quality datasets that provide both positive and negative examples for training. Accessing, normalizing, and preparing the data is a significant challenge today for many organizations.
Technology: While improvements are being made in computing power (quantum and cloud-based approaches), there are still perceived limitations from a user perspective. However, advances in software and user interfaces today remove programming requirements to allow more scientists to utilize machine learning in their research.
Talent shortages: Data science has a well-documented talent shortage, and chemists may not understand how approachable AI is today. Increasing collaboration between chemistry and other scientific disciplines may help accelerate the integration of AI.
An opportunity for growth of machine learning in chemistry
AI and training datasets are being used to solve problems and innovate in scientific institutions across the world, presenting a significant opportunity for data analysis and drug discovery.
Our recent whitepaper has uncovered several areas of chemistry that could benefit from investing in AI technology. The barriers to adoption have never been lower and partners, such as CAS, can help with access to large, quality datasets for analysis. It is possible to solve some of the most pressing problems and take huge strides forward beyond what’s possible with traditional data analysis through the incorporation of artificial intelligence into scientific research.
Find out all about our analysis and the insights we uncovered by reading our whitepaper or contact CAS if you have any questions about how AI technology can support your research.