The boundaries of synthetic chemistry are being pushed to their limits, prompting researchers to look toward biology for solutions. With the rise of synthetic biology and artificial intelligence (AI), scientists are unlocking novel possibilities for drug discovery, such as designing and producing rare and new-to-nature molecules. By harnessing these tools, researchers are developing new approaches to synthesizing complex molecules, optimizing production pathways, and engineering biological systems for pharmaceutical innovation. To gain insight into this rapidly evolving field, we spoke with Graham Hudson, Ph.D., a synthetic biology and microbiology specialist, and Nathan Lanclos, an expert in AI-driven protein design. Learn more about how CAS is tackling these challenges.
CAS: Is synthetic biology a necessary next step for drug development innovation beyond synthetic chemistry?
Theoretically, as long as a structure is chemically sound, synthetic chemistry can access it. But the question isn’t just about feasibility—it’s about efficiency, cost, and scalability. There are compounds that, in theory, you could synthesize, but the effort required to develop a route and the sheer difficulty of controlling selectivity makes it impractical. That’s where synthetic biology can really provide a new avenue for drug synthesis.
Nature has spent millions of years refining enzymatic machinery capable of constructing these complex molecules with remarkable precision, often achieving regio- and stereo-selectivity that can be incredibly difficult to attain with synthetic chemistry. If you take something like paclitaxel, a molecule dense with stereocenters and functionally similar groups, it becomes incredibly difficult to manipulate chemically with the precision necessary to ensure the correct product is formed. But by co-opting nature’s biosynthetic tools, we can take advantage of enzymes that assemble these molecules efficiently.
Additionally, we can take this further with the rise of computational biology and machine learning. AI tools allow us to engineer enzymes beyond what evolution has produced, modifying their structures to catalyze new reactions or expanding their substrate scope. This means we’re not just recreating natural products—we’re opening the door for new-to-nature molecules that could have entirely novel biological properties.
CAS: What recent breakthroughs are helping scientists engineer microbes to be “living drug factories”?
Microbes like Saccharomyces (yeast) and Streptomyces (bacteria) are already widely used to produce valuable compounds, from antibiotics to anticancer agents. The key challenge has been optimizing these systems to produce high yields efficiently. Advances in AI, high-throughput screening, and computational modeling allow us to design and optimize biosynthetic pathways at an unprecedented scale.
We can now use machine learning to predict how metabolic pathways will behave, allowing us to fine-tune enzyme expression levels, optimize precursor availability, and even modify host metabolism to maximize output. For example, it has long been intractable to design experiments on large heteromeric protein complexes to deeply understand long-range protein-protein interactions, but this is now something we are finding ways to do with increasing granularity.
A perfect example of how synthetic biology is transforming drug production is QS-21 (Stimulon, below), an immunostimulatory adjuvant used in vaccines. Historically, QS-21 has been extracted from the soap bark tree (Quillaja saponaria), but the process presents several major challenges. The yields from these trees are incredibly low, making large-scale production environmentally unsustainable and economically impractical. In addition, relying on plant extraction means supply chains are vulnerable to climate variability, deforestation, and geopolitical factors.
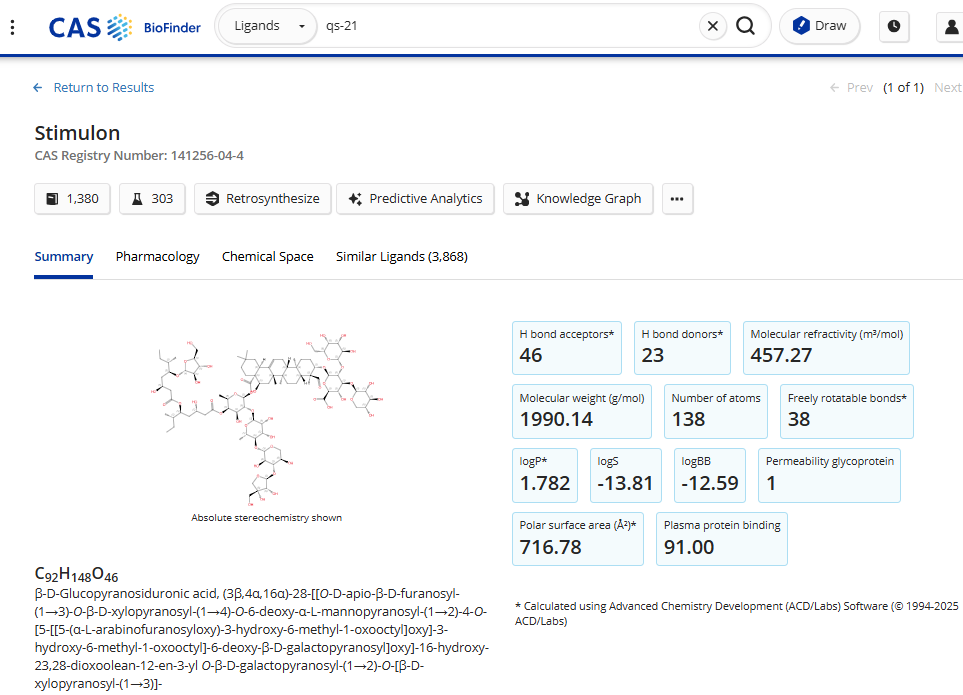
Before we could even attempt to engineer yeast to produce QS-21, we first had to map out its entire biosynthetic pathway and identify the genes responsible for its synthesis. This process was a Herculean effort led by Anne Osbourn’s lab at the John Innes Centre, which involved painstaking transcriptomic analysis across different stages of the plant’s growth cycle.
Unlike microbial biosynthetic gene clusters—which tend to be localized on the genome—plant pathways are dispersed across multiple chromosomes, often with non-coding sequences between the genes. This dispersion made the pathway discovery far more complex, requiring meticulous transcriptomic correlation studies to pinpoint which genes were functionally involved in QS-21 biosynthesis.
CAS: What were the most difficult aspects of adapting plant-derived biosynthetic pathways for microbial production?
One of the biggest challenges was sugar biosynthesis. QS-21 is part of the class of natural products termed saponins, which are complex glycosylated triterpenoids. It has seven different sugars attached to its core structure, but yeast only naturally produces one of them. This meant that, in addition to inserting the biosynthetic pathway for QS-21, we also had to engineer yeast to synthesize non-native sugars.
Another major issue was toxicity. Saponins like QS-21 are membrane-active compounds that can disrupt lipid bilayers and sequester critical native sterols, which is, in part, what makes them effective adjuvants. However, this created a considerable problem in yeast—the QS-21 molecules compromised the integrity of the yeast cell membrane, leading to cell death and production failure. To address this, we had to modify yeast sterol composition to prevent membrane disruption and optimize production conditions.
This entire effort demonstrates the power of synthetic biology. Without these advances, QS-21 production would remain bottlenecked by plant extraction. With engineered bioproduction strains, we now have a scalable, controlled, and sustainable way to produce QS-21. Even though more optimization is needed before large-scale production is viable, this work paves the way for more efficient vaccine formulation.
CAS: In what other areas are you leveraging AI to accelerate discovery?
AI allows us to design and optimize biosynthetic pathways in a way that would be impossible through traditional methods alone. Instead of trial and error, we can use predictive models to determine which enzyme modifications will improve activity, which host strains will be most compatible, and how different pathway components will interact.
For example, in the Keasling Lab, we use AI to engineer polyketide synthases (PKSs), which produce a wide range of bioactive compounds, from antibiotics to anticancer drugs. PKS enzymes are massive, with individual modules containing thousands of amino acids. Their sheer size and complexity make traditional protein engineering approaches incredibly difficult. AI allows us to model these massive proteins, predict how modifications affect function, and design new enzyme variants with improved activity.
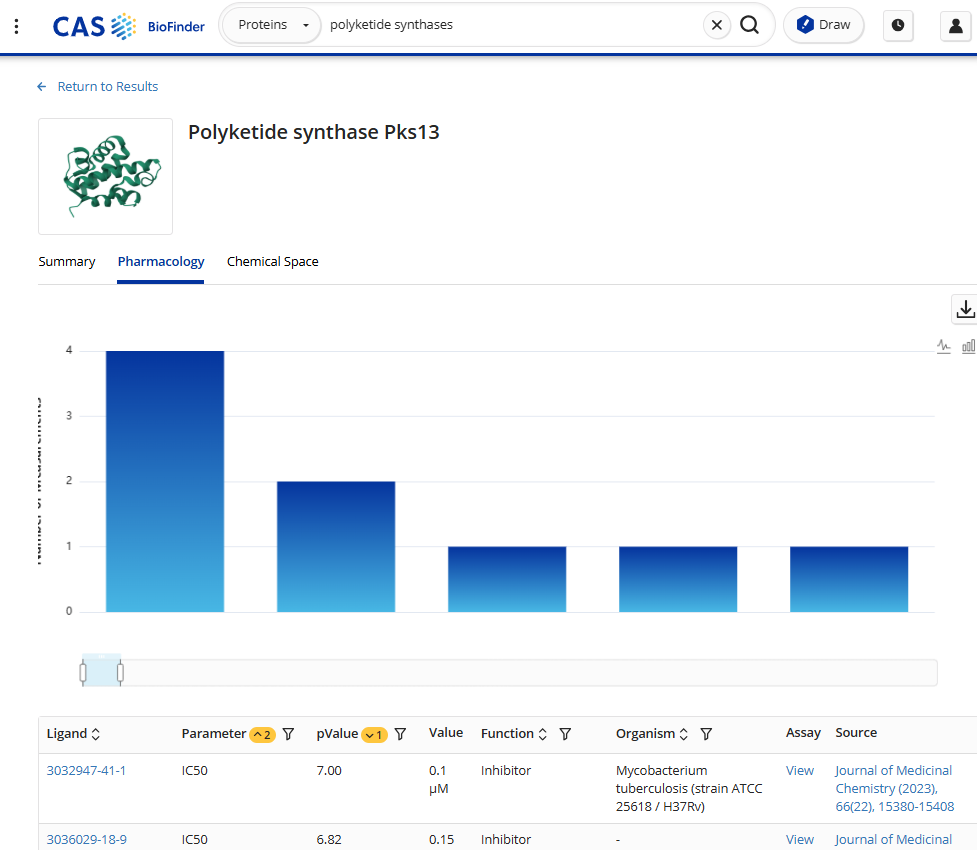
Another area where AI is transforming our approach is enzyme promiscuity and specificity. Enzymes are naturally selective for their substrates, but we often need them to work on non-natural substrates to produce novel derivatives. AI models can help us predict which mutations will broaden enzyme activity without sacrificing efficiency.
CAS: What are some of the biggest data-related bottlenecks you have experienced in your work?
The biggest challenge is data quality and standardization. AI models are only as good as the data we feed them, and historically, biological research hasn’t been structured for large-scale machine learning. Experimental data is often stored in different formats, lacks metadata, or isn’t well-annotated, making it difficult to integrate and analyze at scale.
Currently, too much of my time is spent organizing and cleaning data. A unified data structure for biological, cheminformatics, and AI-generated data would significantly accelerate discovery.
Another issue is instrumentation and data extraction. Many of our analytical techniques—whether LC-MS, HPLC, or GC-MS—still require manual data processing. Instrument manufacturers often use proprietary formats that make it difficult to extract and analyze results automatically. If we had standardized, machine-readable data formats, we could automate a considerable part of the workflow, allowing AI models to learn directly from experimental results.
CAS: Is collaboration between researchers from different backgrounds becoming essential?
At Berkeley, we see first-hand how messy but valuable interdisciplinary collaboration can be. Our bioengineering department includes people from synthetic biology, computer science, hardware engineering, and microfluidics. This diversity allows us to tackle complex problems that no single discipline could solve alone.
One area where this collaboration is critical is in scaling up AI-driven engineering. For example, at JBEI, our microfluidics lab is developing novel devices for optimizing high-throughput strain engineering. Their work enables us to test thousands of different protein designs in engineering cycles that can be as short as a few weeks.
The challenge is that research has historically been siloed. Chemists, biologists, and computer scientists often operate in separate spheres, using different terminology and frameworks. We need better communication and shared knowledge platforms to truly integrate these fields. That alone would massively accelerate progress.
CAS: If you had a magic wand to change anything in the drug discovery process, what would you change?
My answer is a bit philosophical, but I would change how scientific research is valued and funded—especially in biotech. Currently, startups and academic labs operate on a high-risk, high-reward model, where many promising ideas never get fully developed because they don’t fit into traditional funding structures. If we want to unlock the potential of cutting-edge science, we need long-term investment in foundational research, not just short-term profit-driven ventures.
I’d create a system where all prior research was instantly accessible in a structured, machine-readable database. The biggest hurdle we face is that so much valuable data is locked away in PDFs and scattered across different repositories. If we could instantly extract and analyze everything that’s been published on a given pathway, it would accelerate discovery exponentially.
Graham Hudson, Ph.D., earned a B.S. in Biochemistry from Saint Louis University, where he researched RNA base pair thermodynamics to support therapeutic design. He completed a Ph.D. at the University of Illinois at Urbana-Champaign with Douglas A. Mitchell, focusing on RiPP natural products—reconstituting thiopeptide biosynthesis, discovering two novel classes (ranthipeptides and pyritides) and uncovering the enzymatic basis of thioamidation. Currently a postdoctoral researcher at UC Berkeley in Jay D. Keasling’s lab, he studies the enzymology and biosynthesis of saponin natural products. He has also consulted for the industry on natural product research.
Nathan Lanclos holds a B.S. in Molecular Biology and a B.A. in Economics from the University of South Florida, where he researched protein function in cancer with Vladimir Uversky and engineered C1 metabolism in bacteria with Ramon Gonzalez. He is currently a Ph.D. student in the UCSF/UC Berkeley Joint Bioengineering Program. In 2022, he founded a consulting firm supporting biotech startups with marketing strategy and data modeling. His research focuses on machine learning for protein design and high-throughput protein engineering, with an emphasis on multi-domain complexes like polyketide synthases (PKSs) for chemical and therapeutic production.